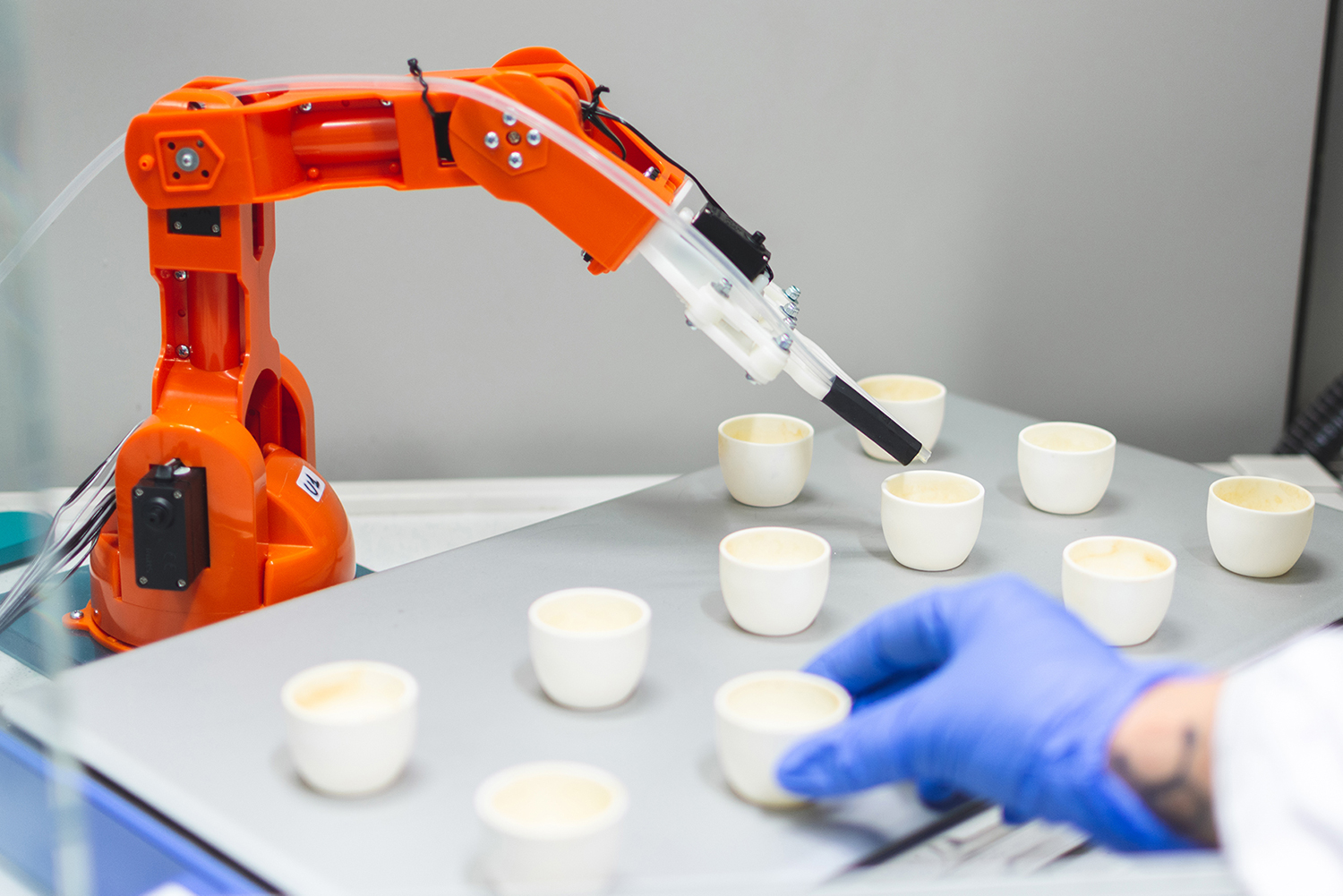
While applications such as electric mobility, stationary storage, drones and medical implants continue to take off, the global demand for sustainable rechargeable batteries is expected to increase drastically in the next decade. Europe alone would need a cell production capacity of at least 200 GWh up to the TWh range.
Li-ion batteries (LIBs) are the most powerful of the high-capacity rechargeable batteries on the market today. This is due, in a big part, to the incessant research and development efforts carried out during the last decades, which have allowed this technology to reach almost their energy density limit. However, this is not enough to satisfy the performance required by many of the new applications such as, for example, the electric vehicle. Therefore, the exploration of new chemical systems that go beyond lithium-ion technology is essential.
In Europe, BATTERY 2030+ is the large-scale collaborative multi-disciplinary research initiative aiming at inventing the sustainable batteries of the future. To address key challenges, BATTERY 2030+ proposes three general themes accross several research areas:
The main limitation of current battery research is that it typically relying on researcher’s chemical intuition and trial-and-error-based development processes. Therefore, the discovery of novel high-performance battery materials and cell designs entails considerable effort, expense, and time - traditionally over ten years from initial discovery to commercialization.
Indeed, the experimental validation stage often is the limiting step of the discovery quest. This process is inherently slow and economically expensive. To mitigate this problem, high-throughput sequencing has emerged over the recent past as a highly efficient approach in many different materials science fields, such as catalysis, polymer and electrolyte research, and biomedicine.
High-throughput experimentation is particularly effective when it is combined with sufficiently accurate theoretical methods in hybrid schemes, where candidate materials are specifically selected for experimental validation upon previous computational screening. However, the applicability of such approaches is limited because of their inherent systematic search of chemical spaces, which often yields a combinatorial explosion and makes impractical the exhaustive rendering of a given candidate space.
Recent efforts to overcome this issue considered moving from pure high-throughput exploration to navigating the candidate space in a selective manner and, by so doing, significantly reduce the number of required experiments.
Essentially, this new paradigm reformulates the traditional discovery process as an optimization problem, where unbiased data-driven algorithms intend to emulate researchers’ chemical intuition. The idea is to implement self-driving laboratories capable of navigating the materials space sequentially using optimization algorithms specifically designed for the application of interest.
In this context, BATTERY2030+ suggests establishing a battery Materials Acceleration Platform (MAP).
Autonomous synthesis robotics, which can be controlled and directed by a central AI, are a central element of this new materials discovery paradigm. Highly automated, high-throughput syntheses are now becoming state-of-the-art for organic and pharmaceutical research, and examples are also emerging in the development of solids and thin-film materials.
For energy storage materials, robotic-assisted synthesis and automation have opened the field to the high-throughput screening of functional electrolytes and active materials constituting anodes and cathodes.
The current limitation is that neither the synthesis protocols nor the characterization are standardized. Since the conditions under which the results are achieved are typically not fully known, the results cannot be fully interpreted and are therefore not universally applicable.
It is generally believed that experimental and computational high-throughput screening of large compound libraries via the use of automation, miniaturized assays, and large-scale data analysis can accelerate materials discovery by up to one order of magnitude with respect to traditional approaches.
Several examples of fully automated high-throughput screening systems for electrolyte formulation, cell assembly, and selected relevant electrochemical measurements are now available, for example, at the MEET Battery Research Center in Germany.
At CIC energiGUNE we have recently started the ION-SELF project, funded by the Spanish Ministry of Science and Innovation and led by Javier Carrasco and Marine Reynaud.
ION-SELF will implement AI techniques feed by first-principles computations and the results of the actual experiments to predict the outcome of new experiments under certain control parameters (composition, temperature, reaction times, reagents, solvent and/or atmosphere, etc.).
The strong link in ION-SELF between theory and experiment will enable, for the first time, to identify consistent correlations among different types of experiments and theoretical calculations. This will bring unprecedented insight into the underlying battery materials discovery process, thereby achieving faster results as compared with brute force high-throughput schemes.
The two main goals of ION-SELF are:
A key aspect of ION-SELF is the feedback for the experiment planner from results of previous experiments, the so-called closed-loop experimentation, which enables the AI-based experiment planner to better hypothesize about the next experiment to be executed.
In order to gear the MAP proposed in ION-SELF, the following two case-studies will serve as proof of concept:
In the last stage of the project, the MAP and the knowledge acquired will be applied to explore other systems, selected from predictive computational tools with the aim to isolate new attractive, disruptive electrode materials.
ION-SELF will help accelerate progress towards a more efficient way of discovering new battery materials, optimizing available resources and increasing success rates of research activities. In a broad context, this should facilitate to achieve the long-term objectives of the European Green Deal.
Javier Carrasco, Group leader of the Modelling and Computational Simulation Research Group at CIC energiGUNE
Oier Lakuntza, postdoctoral researcher of the Modelling and Computational Simulation Research Group at CIC energiGUNE.
If you want to know the latest trends in energy storage and new developments in research, subscribe.
If you want to join a top-level team, collaborate with specialists in multiple disciplines or tell us about your concerns, don't think twice...